Item-Response Theory
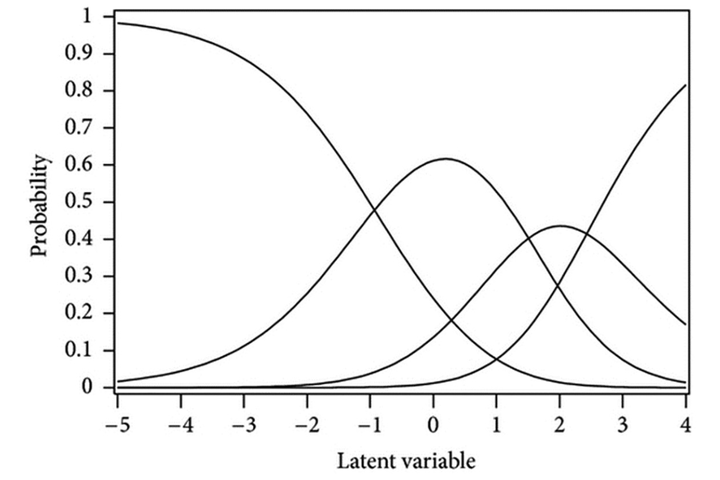
Good day folks, today I would like to make a digression from the usual health economics’ topic addressed on this blog and talk about something different that I have recently taken an interest in. I must apologise for digressing a lot recently but I promise I will cover the topics left back in future posts. It is just that when I would like to talk about something new that interested me I really need to do it as otherwise I will forget it. So, please bear with me when I have these moments.
Of course, we still talk about statistics (what else!) but a kind if statistics to which I have never been exposed to during my student’s years, namely the Item Response Theory (IRT) framework for the analysis of questionnaire data. From my studies I knew the classical test theory consisting of calculating scales’ scores from a given questionnaire or sub-questionnaire items (usually on defined a Likert scale) but I never found the statistics behind this framework particularly appealing. To me it mostly resolved around the use of standard parametric testing methods which do not leave much room for extension from a methodological (particularly modelling) perspective. I do not claim to be an expert in the field and there are certainly ground-breaking development in that statistics’ field too, it is simply something that I did not picture myself working on. In the past I also heard something about IRT as a more sophisticated statistical framework to handle multi-item questionnaires but I never delved into the topic becuase of eithe rlack of time or not the right timing.
Well, perhaps that moment has finally arrived as in the last few weeks I have started reading quite intensively some background theory behind IRT due to some overlap with some consultancy work I had to do for some clients for the analysis of some questionnaire data. Initially I tried to start from the beginning of IRT looking at some old manuals or reference books, such as the Handbook of Modern Item Response Theory from Linden or Fundamentals of Item Response Theory from Hambleton. However, I found these books to be written in a quite old-fashion (which can be quite challenging to read if you know little about the topic or typical methods used in the field) and mostly concerned about detailed explanation of the working behind the algorithm or methods used for estimation in different scenarios. This is certainly useful for someone who already knows what type of model they would like to fit and needs some help on how to implement them, but given my limited knowledge in the field I was quite struggling in following the theoretical motivation and justification for the adoption of such models. In addition the models introduced were quite old and their implementation problems were a result of the limited computational power existing at the time which nowadays can be considerably eased through modern techniques. Finally, none of these references had substantial introduction to IRT from a Bayesian perspective, which is the type of approach I would like to use for such analyses given its flexibility and ease of implementation when dealing with models of high complexity. This is probably due to lack of interest of the authors with such framework and even possibly its limited development from an application perspective of the Bayesian inference due to again computational issues.
Luckily, I have recently discover a very nice and quite recent book called Bayesian Item Response Modeling, from Fox, which nicely summarises the key concepts of IRT from a Bayesian perspective. This is exactly what I was looking for! I have seen that the author from previous IRT manuals was also involved in the writing/giving feedback of the book which is a further hint of reassurance about the quality of the work. I have started reading the first chapters and I really like it so far. The writing is simple and easy to follow and a clear distinction between the more theoretical parts delving into the logic behind the use of such models for handling different types of questionnaire data and the more computational parts where more in-depth explanation about the algorithm used to fit the models are given. I have noticed how the book also goes beyond standard IRT framework with a strong focus on later chapters on multilevel data handling and joint modelling of multivariate outcomes which, for the moment, is not of my interest (but you never know!).
A nice thing about the book is certainly its gentle introduction to IRT which is really appealing to someone like me who only had a vague idea behind the scope of the models, while also providing a quick recap about Bayesian inference (well I did not need that but it is always good to read it again) and some real data examples of most standard modelling approaches with their pros and cons. There are even some snapshots of the old-dear WinBUGS software that was used to fit most of the models (the book is from 1010 so not super recent) with some example codes. Perhaps, if I have to find a negative element so far is precisely the limited amount of software code sessions present across the chapters. I think that a full provision of codes (even just as links to some online repository) would have improved even further the accessibility and appealing of the book to non-statisticians or analysts who would like to try the models themselves but do not know ehre to start with a Bayesian model script.
Regardless, the first few chapters are really nice in terms of providing the background of standard IRT models based on different types of questionnaire data (e.g. binary, ordinal/nominal polytomous, etc…) with some nice explanations on why fitting such models within a Bayesian framework could be beneficial compared to standard classical methods. Here I take some of the author’s words directly from the introduction of the book to make the point:
- The Bayesian modeling framework supports in a natural way extensions of common item response models. The response model parameters are described via prior models at separate levels to account for different sources of uncertainty, complex dependencies, and other sources of information. This flexibility in defining prior models for the item response model parameters is one of the strengths of Bayesian modeling that makes it possible to handle for example more complex sampling designs comprising complex dependency structures.
I completely agree with the statement and I am curious to see in the next chapters how much further the Bayesian potential is covered. From the topics mentioned I can already imagine that, beside an advantage from a computational perspective via MCMC methods, possible reasons will have to deal with the need to account for multilevel structures (e.g. longitudinal or cross-country data) and uncertainty quantification at different hierarchical levels (e.g. person/item level). I thing I am eager to know is the main reason I started the investigation of this topic, i.e. handling of missing item values. Of course, it is well known that such questionnaire data often suffer from missingness problems and it is also quite known (even though often put under the carpet) that standard manuals recommend some sort of ad-hoc imputation in case of partially-observed answers. This is something done to make easier the analysis of total scale scores through standard statistical methods although the implication of such assumptions about the unobserved values is rarely discussed in most cases. I do not expect this to be the main topic of the book but I would be glad to see if this is mentioned anywhere as a possible advantage of Bayesian inference (which I think it is!) since it allows quantification of any unobserved quantity into a model, being it a parameter or missing value. In addition, the use of prior distributions to incorporate missingness assumptions is a powerful tool to assess the robustness of the results of an analysis to a range of plausible assumptions about missingness which standard statistical methodology often struggles with (i.e. some sort of combination of multiple imputation and iterative algorithm to fit the main model - good luck fitting that!).
I think I rumbled enough for today. However, I would certainly talk about this topic more in the future as it is something I am currently have a ton of fun learning and I that I would also like to experiment in my own research at some point (yeah just find the time). Well, till next time!